About Me
I am an AI/ML researcher specializing in medical imaging who is passionate about leveraging deep learning to transform patient care. My expertise centers on developing advanced segmentation models for a range of medical imaging applications, employing cutting-edge techniques to address critical challenges in modern healthcare.
Beyond segmentation, a significant aspect of my research involves enhancing the explainability of AI in medical imaging. I have developed innovative approaches to demystify complex deep learning models, enabling clearer insights into their decision-making processes. This is crucial for ensuring model reliability and uncovering hidden biases, making these tools more transparent and trustworthy for clinical applications.
My commitment to AI in healthcare extends to translating complex algorithms into practical solutions. I strive to bridge the gap between theoretical research and real-world medical applications, focusing on enhancing both the accuracy and understandability of AI tools. This work is vital for building the trust necessary to integrate advanced AI models into clinical practice, ultimately improving patient care and outcomes.
What I'm Doing
Deep Learning
Design and train state-of-the-art models for a wide array of applications.
Medical Segmentation
High-quality segmentation of n‑dimensional medical images.
Computer Vision
Expert image analysis and processing using tools like ITK, VTK, OpenCV, and more!.
Explainable AI
Enabling visual understanding of deep learning models.
Work I'm Proud Of
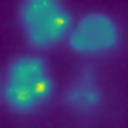
Kernel-weighted contribution: a method of visual attribution for 3D deep learning segmentation in medical imaging
Explaining deep learning model decisions, especially those for medical image segmentation, is a critical step toward the understanding and validation that will enable these powerful tools to see more widespread adoption in healthcare. We introduce kernel-weighted contribution, a visual explanation method for three-dimensional medical image segmentation models that produces accurate and interpretable explanations. Unlike previous attribution methods, kernel-weighted contribution is explicitly designed for medical image segmentation models and assesses feature importance using the relative contribution of each considered activation map to the predicted segmentation.
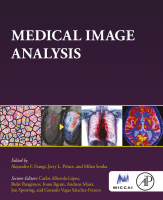
Chapter 18 - Deep learning medical image segmentation
Taking fundamentals of deep learning as pre-requisite, this chapter examines the most relevant approaches to Deep Learning (DL) segmentation starting with Convolutional Neural Networks (CNNs) such as the Fully Convolutional Network (FCN), U-Net, and nnU-Net. Alternative approaches based on Transformer networks are presented. Hybrid Deep Learning and graph-optimization approach Deep LOGISMOS delivers improved performance compared to pure DL methods and allows efficient algorithmic adjudiction. Assisted and sparse annotation approaches are presented that alleviate the burden of manually annotating a large dataset. Explainability of Deep Learning is discussed. The chapter concludes with a case study comparing pure DL and hybrid image segmentation approaches.
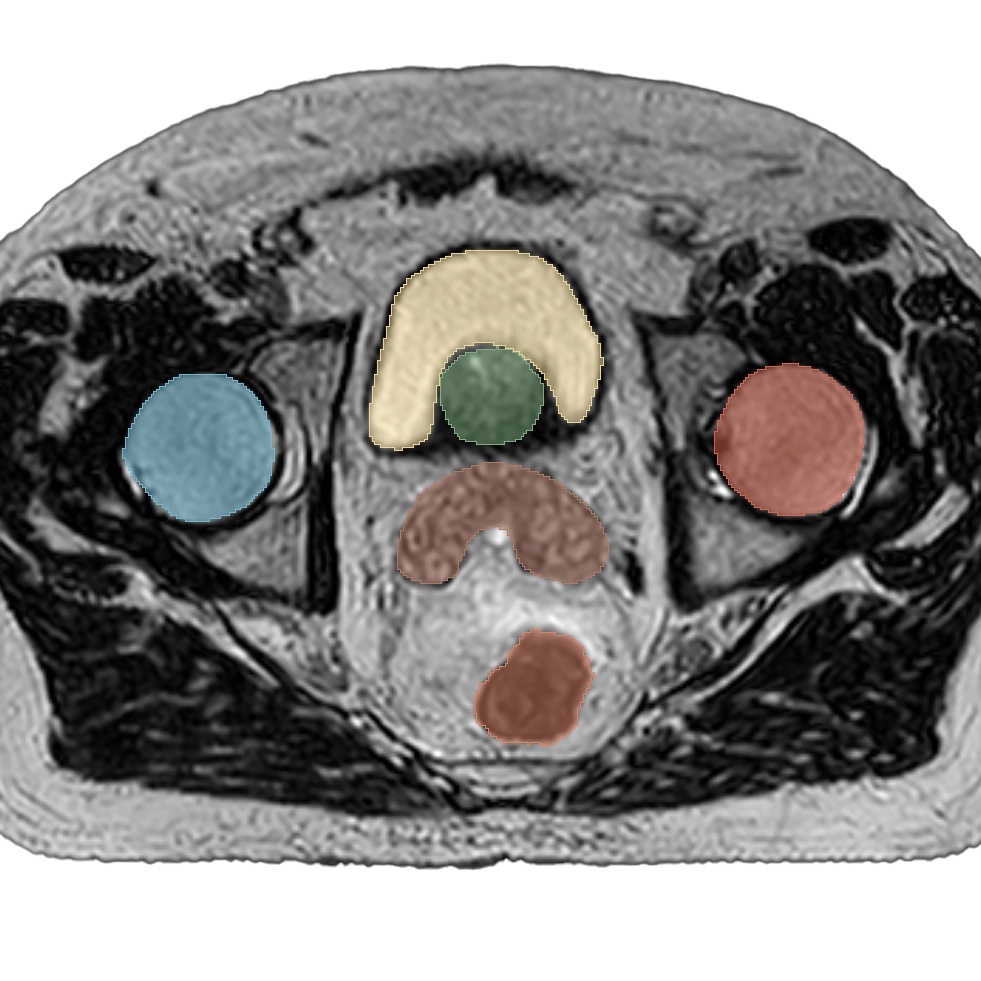
A Technique to Enable Efficient Adaptive Radiation Therapy: Automated Contouring of Prostate and Adjacent Organs
Purpose:
The purpose of this work was to investigate the use of a segmentation approach that could potentially improve the speed and reproducibility of contouring during magnetic resonance-guided adaptive radiation therapy.
Methods and Materials:
The segmentation algorithm was based on a hybrid deep neural network and graph optimization approach that also allows rapid user intervention...
Results:
The absolute accuracy of Deep LOGISMOS + JEI generated contours was evaluated using median absolute surface-to-surface distance which ranged from a minimum of 0.20 mm for the bladder to a maximum of 0.93 mm for the prostate compared with the independent standard across all data sets...Conclusions:
Deep LOGISMOS + JEI segmentation efficiently generated clinically acceptable prostate and normal tissue contours, potentially limiting the need for time intensive manual contouring with each fraction.GoudaMI
Stemming from my general data-analytics python package, Gouda (Good Old Utilities for Data Analysis), GoudaMI is a medical imaging-focused toolkit for deep learning and computer vision tasks. It is designed to be modular and extensible, allowing seamless coordination between ITK, SimpleITK, or NumPy objects through the SmartImage class, which provides a common interface for image processing and analysis. GoudaMI also includes a variety of useful tools for medical image analysis including object analysis, elastic deformation, surface smoothing, vector-aware resampling, and the many many other pieces I've needed for my research over the last decade.